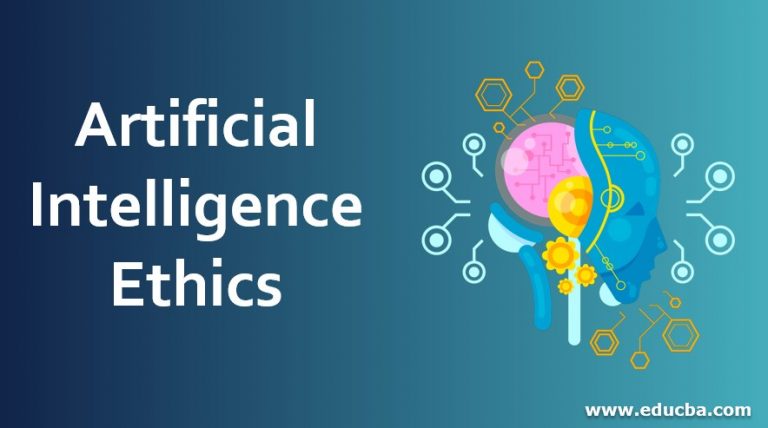
Introduction
Artificial Intelligence (AI) and Machine Learning (ML) are revolutionizing various sectors, from healthcare and finance to transportation and entertainment. As these technologies continue to evolve, so do the ethical dilemmas associated with their use. In 2024, addressing these ethical challenges is crucial for ensuring that AI and ML systems are used responsibly and transparently. This article delves into the key ethical challenges posed by AI and ML, and explores potential solutions to navigate these issues effectively.
The Ethical Landscape of AI and ML
AI and ML technologies are built to analyze data, recognize patterns, and make decisions. While these capabilities offer substantial benefits, they also raise significant ethical concerns, including:
- Bias and Fairness: AI systems can inadvertently perpetuate or even exacerbate existing biases present in the data they are trained on. For instance, facial recognition technologies have been criticized for higher error rates in identifying individuals from minority groups. Ensuring fairness and mitigating bias is a critical concern.
- Privacy: AI systems often require vast amounts of data to function effectively. The collection, storage, and processing of personal data can lead to privacy issues if not handled correctly. Unauthorized data access and misuse can have severe implications for individuals’ privacy.
- Transparency: Many AI systems operate as “black boxes,” meaning their decision-making processes are not easily understood by users. This lack of transparency can hinder accountability and trust in AI systems.
- Autonomy and Control: As AI systems become more autonomous, questions arise about the extent of human control over these technologies. Ensuring that AI systems do not act beyond their intended scope or in ways that conflict with human values is essential.
- Job Displacement: Automation driven by AI and ML has the potential to displace a significant number of jobs. Addressing the social and economic impacts of job displacement is a growing concern.
Challenges in Addressing Ethical Issues
- Data Bias: One of the primary challenges in mitigating bias is ensuring that the data used to train AI models is representative and diverse. Data collection processes must be scrutinized to identify and correct potential biases.
- Privacy Protection: Balancing the need for data to train AI systems with the need to protect individuals’ privacy is challenging. Implementing robust data protection measures and anonymization techniques is vital.
- Algorithmic Transparency: Developing methods to make AI algorithms more interpretable and understandable is an ongoing challenge. Research into explainable AI (XAI) aims to address this issue.
- Regulatory Frameworks: Establishing comprehensive regulations that address the ethical use of AI and ML is complex. Regulatory bodies must work to create guidelines that are adaptable to the rapid advancements in technology.
- Economic Impact: Addressing job displacement requires a multifaceted approach, including reskilling programs and support for affected workers. Collaboration between governments, businesses, and educational institutions is necessary.
Solutions for Ethical AI and ML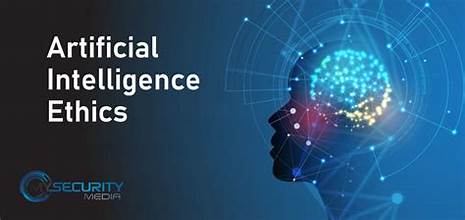
- Implementing Fairness Audits: Regularly conducting fairness audits can help identify and address biases in AI systems. These audits should be carried out by independent third parties to ensure objectivity.
- Enhancing Privacy Measures: Employing techniques such as differential privacy and secure multiparty computation can help protect personal data while still enabling valuable insights. Organizations should also adhere to best practices for data security and privacy.
- Promoting Transparency: Developing and adopting explainable AI techniques can improve transparency. Techniques such as model interpretability and visualization tools can help users understand how AI systems make decisions.
- Establishing Ethical Guidelines: Creating and adhering to ethical guidelines and standards can help organizations navigate the complexities of AI and ML. These guidelines should be developed in consultation with ethicists, technologists, and stakeholders.
- Supporting Workforce Transition: Investing in reskilling and upskilling programs can help workers transition to new roles as AI and ML technologies evolve. Partnerships between industry and educational institutions can facilitate these efforts.
- Encouraging Collaboration: Collaboration between governments, businesses, and academia is crucial for addressing the ethical challenges of AI and ML. Joint efforts can lead to the development of comprehensive policies and best practices.
Conclusion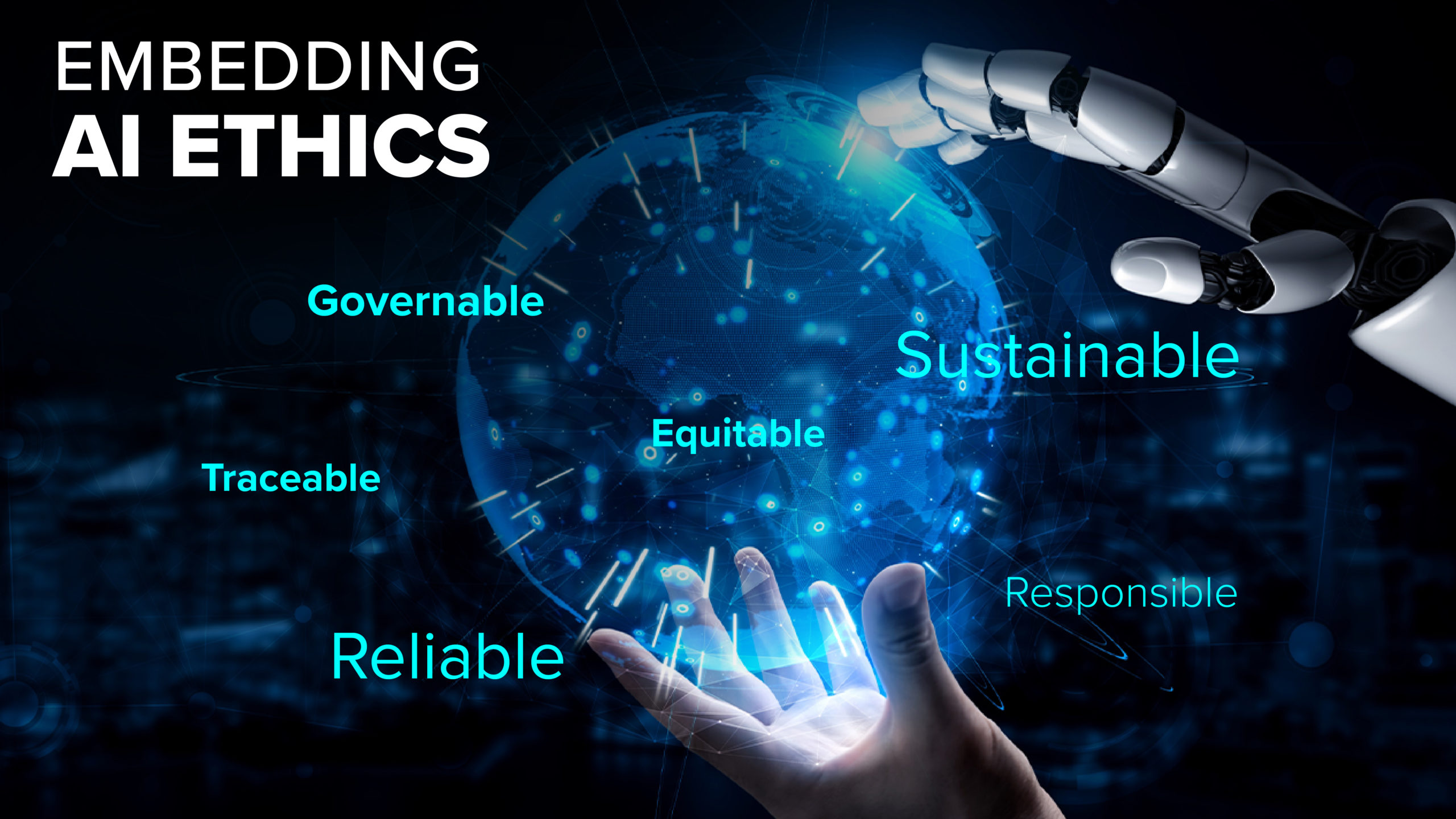
As AI and ML technologies continue to advance, addressing the ethical challenges associated with their use becomes increasingly important. By implementing fairness audits, enhancing privacy measures, promoting transparency, establishing ethical guidelines, supporting workforce transition, and encouraging collaboration, we can navigate the ethical landscape of AI and ML effectively. In 2024, a proactive approach to these challenges will ensure that AI and ML technologies are used in ways that are ethical, fair, and beneficial to society.